ANALYSIs: STRATEGY - predictive models in online crypto casino & sportsbook
- Storz Pawel
- Nov 1, 2024
- 2 min read
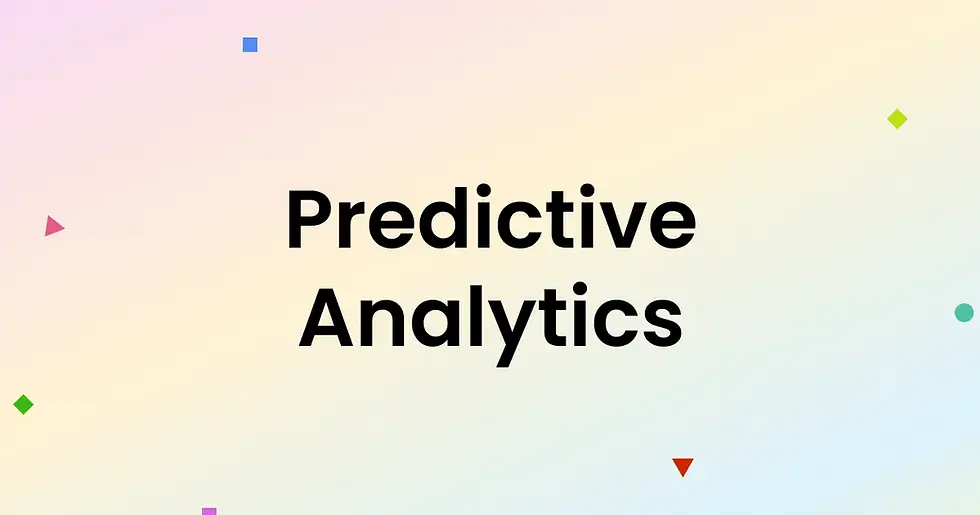
Using predictive models differs from traditional Key Performance Indicators (KPIs) primarily in terms of objectives, data processing methods, and application of results. Both approaches aim to optimize and improve business operations, but predictive modeling provides much more advanced insights that allow for forecasting future events, rather than merely evaluating past performance.
The key differences between predictive models and KPIs:
Objective and Scope of Analysis
KPIs: Primarily used to monitor performance in specific business areas. KPIs indicate the current state (e.g., current revenue, customer retention, conversion) and show whether the company is meeting its business goals in various areas.
Predictive Models: Their main goal is forecasting future events and identifying patterns, enabling the company to make decisions based on predictions (e.g., forecasting customer churn, predicting sports betting outcomes). These models can reveal the probability of events that KPIs cannot directly measure.
Data Processing – Past vs. Future
KPIs: Analyze historical and current data to assess the present state or performance over the past period. They rely on straightforward metrics (e.g., the number of active users, win-to-loss ratio, revenue per user) and have a retrospective application.
Predictive Models: Based on advanced statistical analysis and machine learning, predictive models can process large amounts of historical data, but their goal is forward-looking – predicting trends, risks, or outcomes based on patterns identified in the data. In iGaming, this could mean predicting how a specific player segment will behave or which games will become popular.
Flexibility and Application of Results
KPIs: Metrics are simple and direct, allowing for quick monitoring of results, but they have limited flexibility in forecasting future actions. They can only indicate where changes are needed but do not provide guidance on how to implement those changes or predict their outcomes.
Predictive Models: Applying predictions in strategic decisions provides flexibility – enabling "what-if" scenario analysis and planning of various courses of action. In iGaming, these models can predict, for example, the impact of different bonuses on a specific customer segment or the risk associated with different sportsbook odds levels.
Context and Complexity
KPIs: Are straightforward point indicators and relatively simple. KPIs measure specific aspects, offering a clear picture of results but limiting context. In iGaming, KPIs can show, for instance, monthly revenue or conversion rate but will not explain the reasons for changes or provide forecasts for future changes.
Predictive Models: Based on more complex modeling and data analysis, they offer a broader perspective, taking into account multiple variables simultaneously. In iGaming, this allows the company to analyze relationships between different variables, such as seasonality and the number of active players, which may not be visible in KPIs.
KPIs help in monitoring and evaluating past performance, while predictive models support forecasting and decision-making for the future. iGaming companies, by leveraging predictions, can better plan retention strategies, anticipate player behavior, minimize risk, and adapt their actions to anticipated market changes, allowing them to respond more effectively to industry needs and trends.
Comments